by S. Kazem Banihashem, Open University, the Netherlands.
The future of education is becoming increasingly entwined with Artificial Intelligence (AI). The integration of AI into education is an inevitable trajectory that is fundamentally reshaping the landscape of learning for the future. Students are already and swiftly integrating AI tools like ChatGPT and Gemini for various purposes, including generating learning materials, facilitating writing tasks, and providing feedback. However, research shows that students often rely on AI as a shortcut, rather than using it as a tool for deeper learning. Such dependency may inhibit the growth of key abilities like self-regulation, sparking concerns about students’ ability to regulate their learning in the age of AI.
This concern has paved the way for a new research frontier at the intersection of AI and Self-Regulated Learning (SRL). Researchers in this emerging field are increasingly examining key issues, such as how to effectively guide and regulate students’ learning in an AI-driven education sector, and how AI can be harnessed to support and enhance self-regulation skills. They are also investigating the theoretical foundations behind AI’s role in SRL contexts. By exploring these dimensions, AI-SRL research seeks to develop frameworks and tools that position AI not just as a passive resource, but as an active partner in fostering effective, independent learning.
In our work, we set out to systematically map the expanding literature on AI-SRL research, aiming to highlight AI’s contributions to SRL, identify existing challenges and gaps, and outline future directions for AI-SRL research.
Approach
We employed a systematic mapping review, adhering to the PRISMA method, to categorise and synthesise existing research at the AI-SRL intersection. The review was guided by the framework of Chatti et al., which explores four key dimensions of educational research: Who (the stakeholders), What (theories and models), How (the methods used), and Why (the objectives of AI interventions).
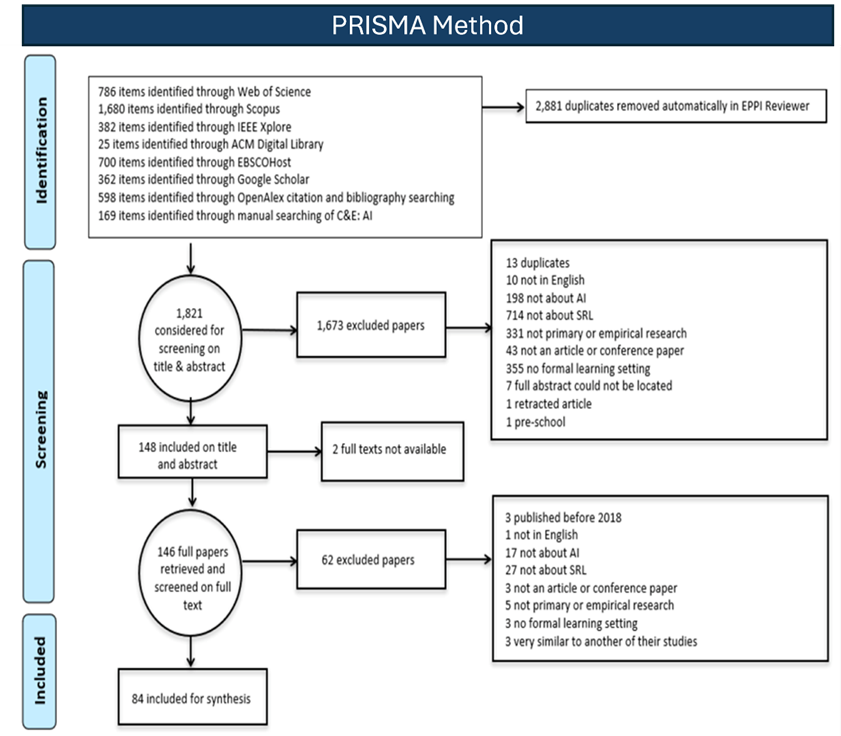
Findings
- Focus on Higher Education, Limited K-12 Research. Most AI-SRL studies focus on higher education, with little research on K-12 students. Given the importance of early development in self-regulated learning skills, this gap needs addressing.
- Lack of Theoretical Foundations. Over one-third of AI-SRL studies lack a solid theoretical foundation, making it difficult to evaluate their effectiveness and apply findings across diverse educational contexts.
- AI’s Role in Adaptive Learning and Personalisation. AI in SRL is mainly used for adaptive learning and personalisation, tailoring content and feedback to individual student needs and predicting learning behaviours.
- Emphasis on Cognitive Skills, Motivation Overlooked. While AI-SRL studies focus heavily on cognitive and metacognitive skills, motivation—a critical component for long-term learning engagement—is often neglected.
- Geographic Focus on Certain Regions. AI-SRL research is concentrated in North America, Asia, and Europe, with limited studies from regions like the Middle East and Africa, leaving important regional contexts unexplored.
- AI’s Broader Impact Beyond SRL. AI’s role extends beyond SRL, helping improve academic performance, motivation, and engagement, offering real-time feedback and enhancing overall learning experiences.
Key Takeaways
Longer-Term Studies Needed: Many of the studies were short-term, making it difficult to assess the sustained impact of AI on SRL. Longitudinal research is needed to evaluate long-term effectiveness.
Focus on Motivation: Motivational aspects of SRL, such as self-efficacy and persistence, are underexplored. Future research should address how AI can be designed to enhance motivation, a crucial factor for sustained learning success.
Ethical and Pedagogical Standards: Educators must ensure that AI interventions adhere to ethical standards, including transparency, student consent, and data security. Involving educators in the design of AI tools is essential to align these tools with classroom needs.
Broader Use of AI Tools to Foster SRL Development. AI tools like chatbots and predictive models hold promise for addressing diverse learning needs and identifying at-risk students. However, to ensure students develop SRL skills rather than relying on AI for quick answers, these tools should actively promote self-regulation by guiding students through the learning process, encouraging reflection, and fostering independent problem-solving.
This article was adapted from: Banihashem, S. K., Bond, M., Bergdahl, N., Khosravi, H., & Noroozi, O. A Systematic Mapping Review at the Intersection of Artificial Intelligence and Self-Regulated Learning: A call for increased theoretical grounding, focus on motivation and diversifying context. Preprint. https://tinyurl.com/AI-SRL-Review
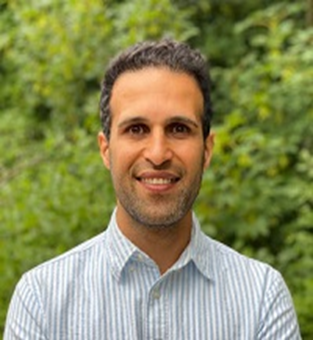
Seyyed Kazem Banihashem (Open University, the Netherlands)
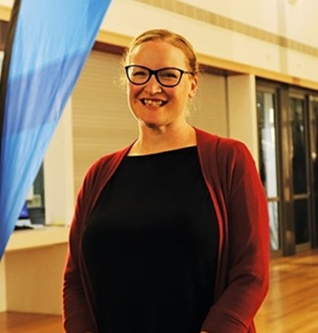
Melissa Bond (University College London, UK; University of Stavanger, Norway)
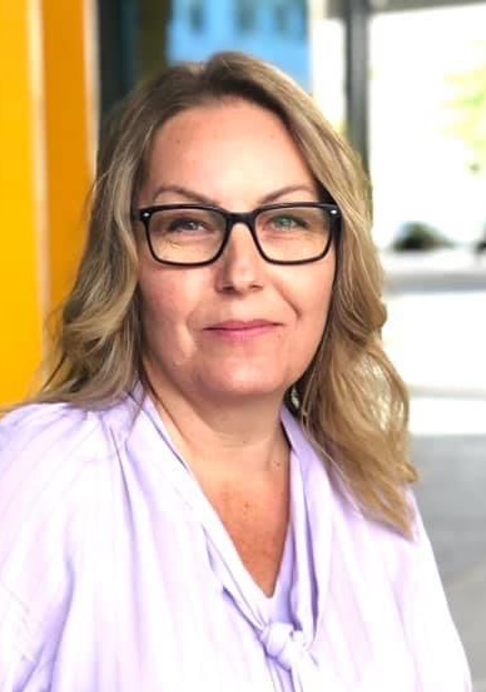
Nina Bergdahl (Halmstad University, Stockholm University, Sweden)
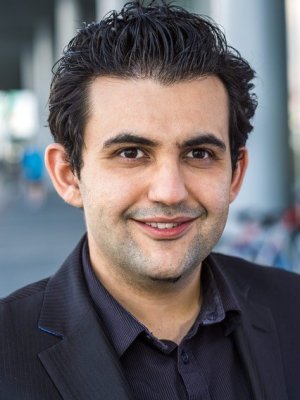
Hassan Khosravi (The University of Queensland, Australia)
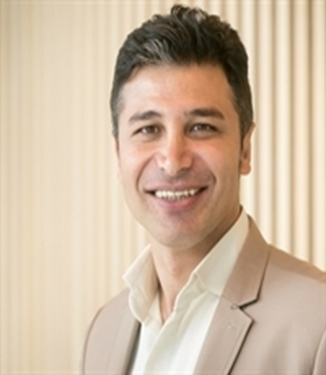
Omid Noroozi (Wageningen University and Research, The Netherlands)
*Feature image – Copilot-Generated Photo