by Helen Nicholson, Jisc, UK.
Automating transcriptions and captions is perhaps one of the most widespread uses of artificial intelligence (AI) in education today.
When aiming to increase accessibility, these technologies— which frequently combine Automatic Speech Recognition (ASR) and Natural Language Processing (NLP) to produce text from audio —are a key focus. Indeed, the provision of captions for pre-recorded materials is a requirement of the 2018 Public Sector Bodies Accessibility Regulations (PSBAR). Furthermore, our most recent student digital experiences insights survey found that 29% of HE students used captions or transcripts to support their learning.
At this time though, the Web Content Accessibility Guidelines (WCAG), which PSBAR require institutions to meet, state that automatically generated transcripts and captions are not accurate enough and must be edited by a human to be compliant. Advances in ASR technology are eagerly awaited as the burden of manually editing transcripts, or paying for professional transcription services, puts widespread captioning of educational materials out of reach for many institutions.
Like many AI assisted technologies, keeping track of the advances in ASR can be difficult. To better understand the current situation this article will look at the limitations of ASR tools today, what’s different about new releases like Whisper from OpenAI (the company behind GPT-3 and DALL.E 2) and, what this might mean for the future of transcription tools.
Human parity?
Many companies have focused on human parity as a kind of end goal for ASR – seeing how the model can compare to a human transcriber on the same set of data.
Microsoft already claimed to have achieved human parity in 2017 with their ASR model. However, there has been criticism of this claim as the model was tested using a benchmark test, Switchboard, which contained clear, high quality audio data primarily from native English speakers. This kind of benchmark doesn’t account for the wide variety of speech and audio conditions that an actual human transcriber might handle.
This is the primary issue of ASR tools today – they struggle with the multitude of variations in human speech, therefore accuracy of these tools falls significantly when they encounter less common situations, such as:
- Low quality audio equipment
- Multiple Languages
- Multiple speakers
- Background noise
- Varied accents, dialects and non-standard speech
- Ranging vocabulary
Achieving a recording which avoids these issues entirely would be impossible, particularly in an educational context. A recording in a busy lecture theatre will inevitably have background noise, a Chemistry seminar will have specialist terms, and it is impractical to expect every classroom to be kitted out with top quality audio equipment.
There is a larger accessibility issue at play here as well; these tools are inherently biased against groups who already experience access issues in UK higher education, such as disabled people and non-English speakers, because they work best with “standard” audio, which is primarily from native, non-disabled English speakers.
Advances are being made to address these issues in order to create tools which can provide accurate transcripts for more diverse audio. This is where OpenAI’s Whisper comes in. This model, rather than claiming parity with humans, claims to be “approaching human levels of robustness”. Robustness is key to the future of ASR as this is that ability of the model to perform well across different situations, in this case across that wider variety of speech.
Whisper
The primary difference with Whisper is in its training data which comprises a much larger, more diverse dataset of audio than typical ASR models.
Whisper has been trained on 680,000 hours of audio data – this is magnitudes away from other ASR models which may be trained on far less than 10,000 hours. Further, around 1/3 of the dataset is not in English, and this non-English data also comprises audio from over 98 other languages.
Whisper does not yet beat other models in some commonly used performance tests, OpenAI explain that this is because of the diversity of its dataset rather than in spite of it. Where it does excel is in tests of robustness, in tests across diverse datasets Whisper made 50% fewer mistakes than existing ASR models. OpenAI have demonstrated that increasing diversity in the training data can improve a models ability to handle accents, multiple languages, background noise and wider vocabularies.
This is incredibly promising for ASR and accessibility, though there are caveats to Whisper currently. OpenAI have sourced Whisper’s dataset from the internet in much the same way to how they source mass amounts of images and text for DALL.E 2 and GPT-3. This makes the scale of the dataset possible without it costing an exorbitant amount to create, however it can cause the model to produce hallucinations. In this case hallucinations are text that is not at all related to the audio provided. These mistakes can be quite jarring too – a compilation of these from Twitter user Ryan Hileman shows the model transcribing rambling, incoherent paragraphs from single word audio clips.
The developers of Whisper also acknowledge that the model is still lacking on many languages, the data has been sourced from English focused parts of the internet which still results in a bias towards English. There are also indications from Whisper that increasing the dataset in this way can only go so far to improve the model, they note that the increase in English language performance begins to diminish noticeably between 13,000 and 54,000 hours of data.
Ongoing efforts
Importantly, Whisper isn’t the only effort to create new and inclusive datasets nor is this the only way we will see ASR improve in the coming years.
There are several ongoing efforts to crowdsource voice clips in order to achieve diverse data without the issues that arise from internet scraping. You can explore and even contribute to many of these online:
- Check out Project Ensemble, an initiative run by Voiceitt to create a data set of non-typical speech. Project Ensemble is part of larger work, The Nuvoic Project, being conducted by Voiceitt with partners, The Karten Network, to develop an application that can process non-standard speech continuously.
- Mozilla’s Common Voice project is another seeking to create a large data set which represents voices traditionally excluded from the most commonly used data sets including non-English speakers, disabled people and LGBTQIA+ people.
Overall, considerable work is going toward remediating the limitations of ASR technology and we should see more accurate, inclusive and useful ASR tools in the coming years that hopefully can alleviate a lot of the difficulties around transcription in education. We cannot predict when or if automatic tools will reach a level that can meet standards like WCAG but the outlook is promising.
In the meantime, our Accessibility community is a great place to discuss issues and solutions around transcription and other accessibility concerns.
Find out more by visiting our National centre for AI page to view publications and resources, join their events and discover what AI has to offer through our range of interactive online demos.
Editor’s note: This article was first published on Jisc’s blog.
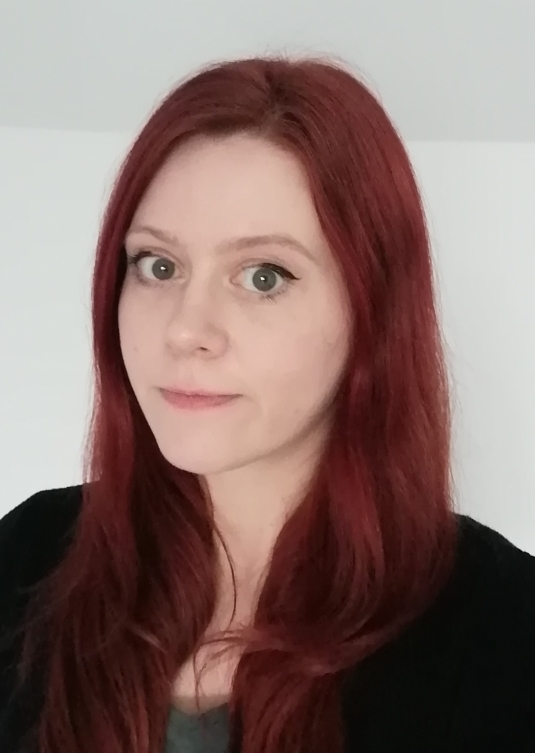
Author
Helen Nicholson, Jisc, UK