People with a hearing impairment can benefit from captioning of speech and sounds in videos and people with a visual impairment can benefit from audio description of images and actions in videos. Captioning and audio description can also be useful for anyone unable to hear or see a video for environmental reasons (e.g. sound to quiet or too much background noise or vision is obscured). This article discusses how speech recognition captioning with collaborative editing can provide affordable transcription/captioning of lecture recordings and educational videos and discusses the potential of Artificial Intelligence for audio description and sign language translation.
Many countries have had long standing regulations about captioning or audio description of broadcasted television programs but these don’t normally apply to online video. The UK’s Equality Act 2010 requires universities to make anticipatory reasonable adjustments and so universities should caption all their lecture recordings rather than only caption a lecture recording if requested by a deaf student.Paying captioning companies commercial rates for captioning lecture recordings is not affordable by universities but automatic speech recognition captioning is affordable.
One of the arguments that some of the captioning companies use to persuade customers to use their manual captioning service is to say that speech recognition automatic captioning although much cheaper, produces inaccurate captions. Although background noise and music and poorly positioned microphones can reduce the accuracy of automatic speech recognition, it can be as accurate as human transcribers, especially if students are used to collaboratively correct caption errors.
Unfortunately no lecture capture company’s technology currently enables collaborative editing.
Synote was developed to enhance learning from lecture recordings through collaborative editing of speech recognition transcription with additional features including synchronised notes, clip playlists and a print friendly audio only version as shown in the following screen captures.
It is important for teachers to make a good quality recording and this can be achieved by wearing a wireless microphone and adjusting the recording level to provide a good signal to noise ratio. If a teacher uses a fixed lectern microphone and turns or moves away from the microphone to write on the board or walk around the room then the recorded speech level and signal to noise ratio will decrease. If the lecturer repeats any questions or comments or answers from the students then the speech of the students does not need to be transcribed. It is also possible to record and transcribe the speech of the students using a wireless microphone, either handheld or passed around or using an app on a mobile phone.
As the quality of the recording worsens then speech recognition may struggle more than human transcribers and a solution to this problem of improving the accuracy of speech recognition transcription is to use students to collaboratively correct errors. Students correcting errors engage more strongly with the lecture content to improve their learning and so justifying the awarding of academic credit for correcting errors. Universities and students could choose appropriate rewards.
Students correcting errors in their own lectures involves little extra effort while they listen, watch, and read the recording and captions as it is difficult not to notice errors. They also generally know the subject better than a professional captioner as captioning companies do not guarantee to provide a specialist in that subject. Images and slides can be automatically synchronised with the transcript to enable printing out of all of the information from a lecture.
Artificial intelligence machine learning using Deep Neural Networks has proved very powerful for improving the accuracy of speech recognition and recognising images. AI can also be used for enhancing speech recognition through automatic lipreading and can also recognise some sounds on videos for captioning (e.g. Applause, music and laughter). AI can also modify lip patterns and faces on videos to make them easier to lipread.
AI cannot however currently provide automatic audio description of videos as this requires reasoning and understanding subtle meanings and context to identify what visual information is important. For example in a video if a person leaves the room then while it might be possible for the AI system to identify this had happened this might only be important information to provide in an audio description if it was necessary for the viewer to know that the person leaving the room could not have heard what was being discussed in the room after they had left the room.
AI can also provide automatic sign language translation of the captions using avatars although the quality of translation for a visual language is not as good as translations between written languages which have vast amounts of data available for training the AI systems.
Mike is one of the keynote speakers at the Media & Learning Conference on Video in High Education and will be speaking on the topic of accessibility on 6 June in Leuven.
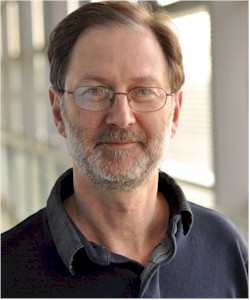
Author
Prof. Mike Wald, University of Southampton, UK
Mike’s background
More about Mike’s research activities. Mike will give a keynote presentation at the Media & Learning Video in Higher Education Conference on 5-6 June 2019