By Dr Gert Vanthournout, Yasmine Wauthier, Dr Silvia Van Aken, Kristof Overdulve & Lowie Spriet
There is an enormous potential for the use of Virtual Reality (VR) in training. This seems especially true for so-called complex skills (or problem solving), where trainees must combine ‘thinking’ with ‘doing’, sometimes under time pressure. Frequently mentioned advantages include safety, durability and affordability. But VR can also increase the return on investment (ROI) of training, compared to traditional training formats, although this added value up till now remains somewhat under exposed and under researched. The project “AI-driven VR training in an adaptive user context”, funded by the Flemish Agency for Innovation & Entrepreneurship, is a collaboration between the AP University of Applied Sciences and Arts, and the University of Antwerp (Belgium). It explores how the deliberate use of (1) an educational design model, (2) Artificial Intelligence (AI) and (3) 3D scanning techniques which can improve the ROI of VR training.
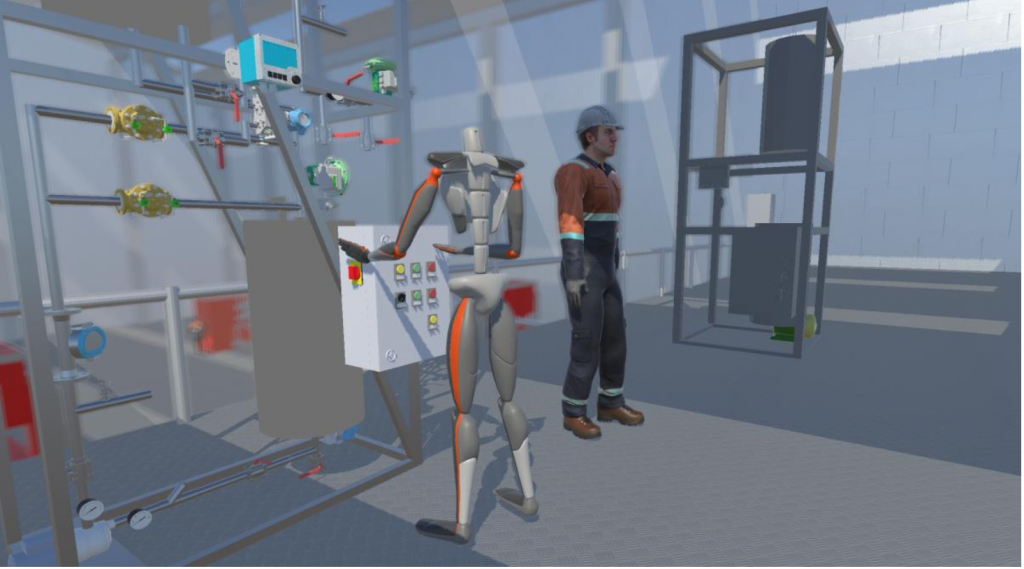
POC 1 – Adaptive gas analysis VR training (AP University of Applied Sciences and Arts).
The focus of research concerning VR training is often primarily on the technical development of the training environment, relocating educational design to merely an afterthought. Training environments are developed ‘intuitively’ or based on blueprints for traditional training formats. In our project we hypothesize that an explicit educational model will increase the quality of the training environment, resulting in a higher ROI. As we did not find a model that suited our needs, we decided to develop it ourselves. Rather than starting from scratch, we based ourselves on existing educational models including the 4 Component Instruction Design Model (4CID), Zimmerman’s model for self-regulated learning (SRL), and Ryan and Deci’s self-determination theory (SDT). We integrated and applied insights from these frameworks into a 7-step operational model, we called it the Instructional Model for Immersive Learning (IMIL). This model provides a roadmap on how to guide trainees towards independently executing complex tasks and problem solving and is used as a blueprint for developing the two Proof of Concept (POC) Training Experiments in our project.
Our project also investigates the possibilities and challenges of Artificial Intelligence (AI) to improve the return on investment (ROI) of VR training. The potential here lies within the concept of adaptive training: the better a training is adapted to the needs of a trainee, the higher the expected ROI. For trainers it is often challenging to fit the pace and level of difficulty to that of trainees, especially in group-based formats. Training programs can be custom tailored by changing the order of scenarios or by making it more easy or difficult. In our architecture, training scenarios can be configured and enriched by so called complications that determine the difficulty of certain tasks and simplifiers that determine how much the trainee is assisted in completing tasks through e.g., virtual agents. Through gamification, we estimate the competence level of the trainee and generate custom tailored scenarios automatically.
AI algorithms can not only custom tailor training scenarios to the individual, but also improve the workflow of creating immersive VR training. If we want trainees to transfer what they learn in the VR training to their actual workplace, and thus improve the ROI, it is important that the training environment sufficiently resembles the working environment. However, creating such a detailed 3D environment is time and energy consuming. Advanced 3D scanning techniques might help reduce these costs. Unfortunately, currently 3D scanners only generate static geometric point clouds that need thorough postprocessing to apply them in interactive virtual environments. We aim to improve the workflow of 3D scanning by automatically segmenting a point cloud into individual objects – classifying points as belonging to a chair, wall or floor – and turning the point cloud into polygonal meshes, into which realistic physics can be applied.
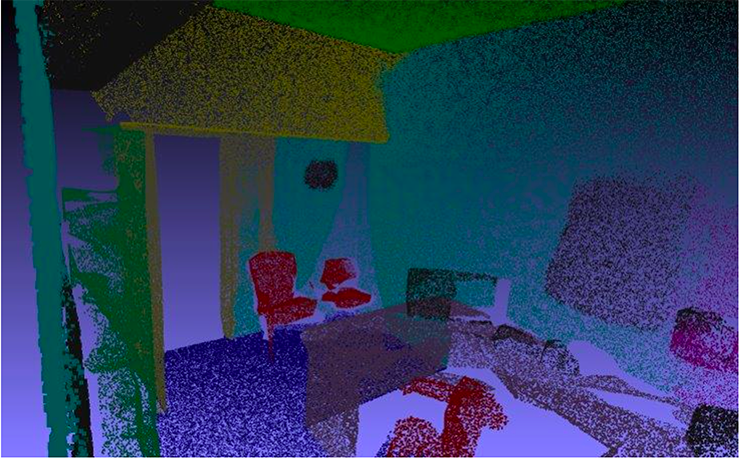
POC 2 – VR Safety training: we aim to automatically segment a point cloud into individual objects and polygonal meshes, into which realistic physics can be applied.
As this project will finish in March 2023, results remain preliminary. An initial version of the IMIL model is available in addition to the first batch of 3D scans. The latter however still need to be integrated in the actual training environment. Moreover, the development of two POC Experiments is still ongoing. Testing with real live trainees is set for June 2022 at the earliest. Additional results regarding trainees’ perceptions and ROI of the training are expected from November 2022.
Authors
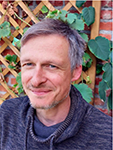
Dr. Gert Vanthournout, AP University of Applied Sciences and Arts, Data-driven Learning & Innovation, Belgium.
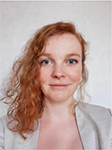
Yasmine Wauthier, AP University of Applied Sciences and Arts, Immersive Lab / Data-driven Learning & Innovation, Belgium.
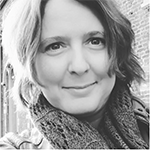
Dr Silvia Van Aken, AP University of Applied Sciences and Arts, Immersive Lab, Belgium.
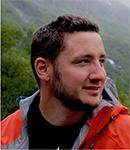
Kristof Overdulve, AP University of Applied Sciences and Arts, Immersive Lab, Belgium.
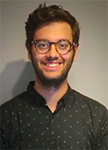
Lowie Spriet, AP University of Applied Sciences and Arts, Immersive Lab, Belgium.